Rethinking Incarceration and Reentry: A Q&A with Emily Owens
Rethinking Incarceration and Reentry: A Q&A with Emily Owens
Three takeaways on Bipartisanship from Punchbowl’s New Power Players

Studying Restorative Justice in Schools: A Q&A with Anjali Adukia and Benjamin Feigenberg
Community Safety
Studying Restorative Justice in Schools: A Q&A with Anjali Adukia and Benjamin Feigenberg
Q&A

Promising Evidence on Youth Violence Reduction Programs: A Q&A with Nour Abdul-Razzak
Community Safety
Promising Evidence on Youth Violence Reduction Programs: A Q&A with Nour Abdul-Razzak
Q&A
Commercial Sector Prices
Medicare Already Pays 2 – 4x More for the Same Care — and the Gap is Growing
Our 2023 Approach to Giving
Infrastructure
Fixing America’s Housing Crisis: A Blueprint for Change

Can “Procedural Justice” Help Police Boost Morale and Improve Public Trust?: A Q&A with Karen L. Amendola and Colby Dolly
Community Safety
Can “Procedural Justice” Help Police Boost Morale and Improve Public Trust?: A Q&A with Karen L. Amendola and Colby Dolly
Q&A

Studying Sentencing Reforms: A Q&A with Steven Raphael
Community Safety
Studying Sentencing Reforms: A Q&A with Steven Raphael
Q&A
Higher Education Quality and Accountability
Connecting Education & Opportunity: A Framework for Credential Impact in Tennessee

Video: The Day One Campaign: Making the Pretrial System More Effective, Speedy, and Just
Pretrial Justice
Video: The Day One Campaign: Making the Pretrial System More Effective, Speedy, and Just
Video

“Housing is a gateway to all the other things in people's lives.”
Affordable Housing
“Housing is a gateway to all the other things in people’s lives.”
Q&A
Community Safety
Studying the Effects of Youth Violence Reduction Programs: A Q&A with Becky Pettit, J. Mark Eddy, and Shana Fox
Q&A

Video: Bridging the Gap Between Research and Policy: A Q&A with Elizabeth Glazer of Vital City
Criminal Justice
Video: Bridging the Gap Between Research and Policy: A Q&A with Elizabeth Glazer of Vital City
Video

Why You Should Beware Hospital Consolidation
Commercial Sector Prices
Why You Should Beware Hospital Consolidation
Op-Ed
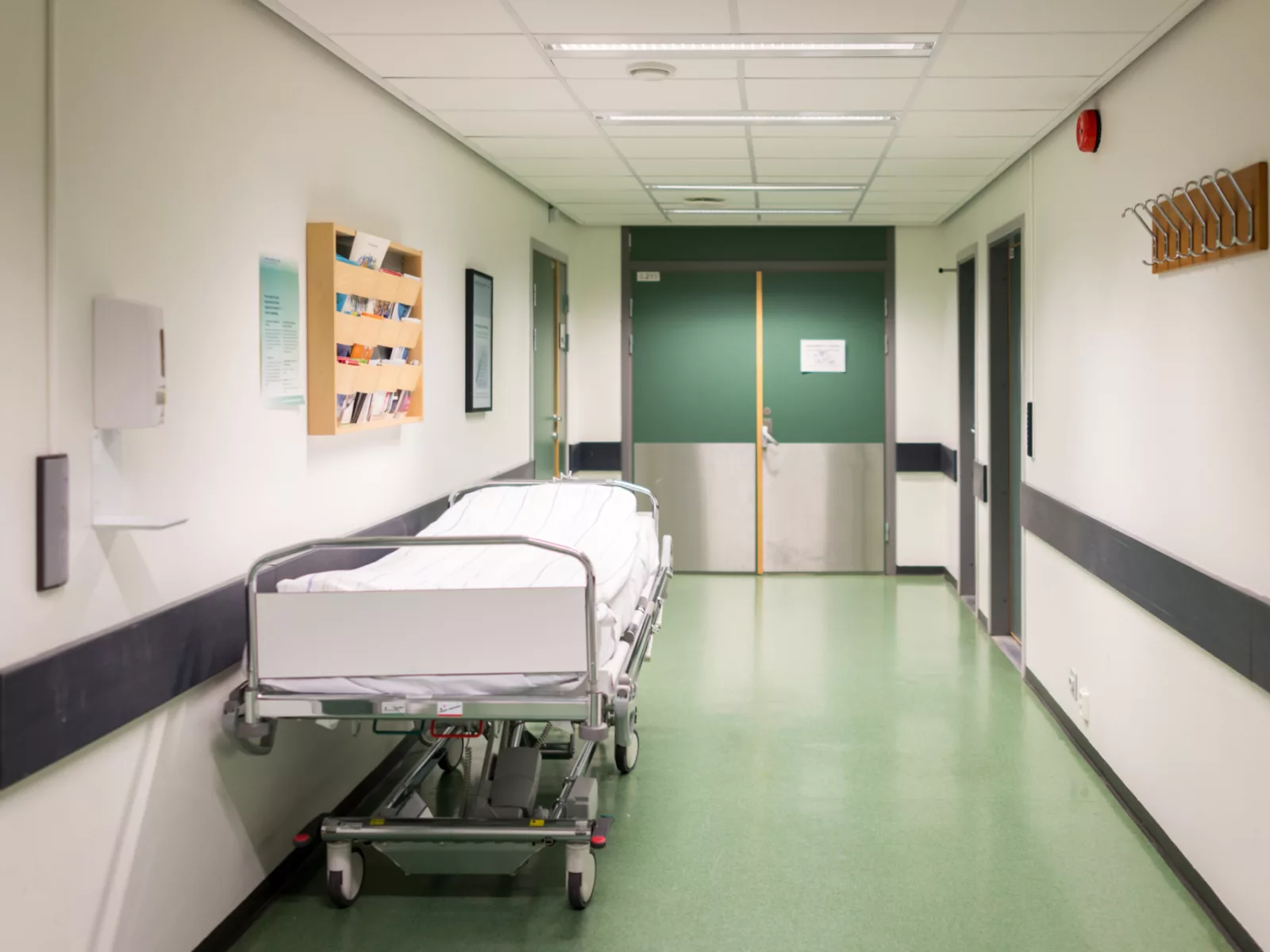
Time for a Shift in How We Pay for Primary Care
Provider Payment Incentives
Time for a Shift in How We Pay for Primary Care

Could AI Help Make Prosecutors’ Decisions Race-Blind? A Q&A with Alex Chohlas-Wood
Prosecution
Could AI Help Make Prosecutors’ Decisions Race-Blind? A Q&A with Alex Chohlas-Wood
Q&A

“Not a Fair and Open Market” John Arnold on Health Care Reform at Forbes 400 Philanthropy Summit
Drug Prices
“Not a Fair and Open Market” John Arnold on Health Care Reform at Forbes 400 Philanthropy Summit
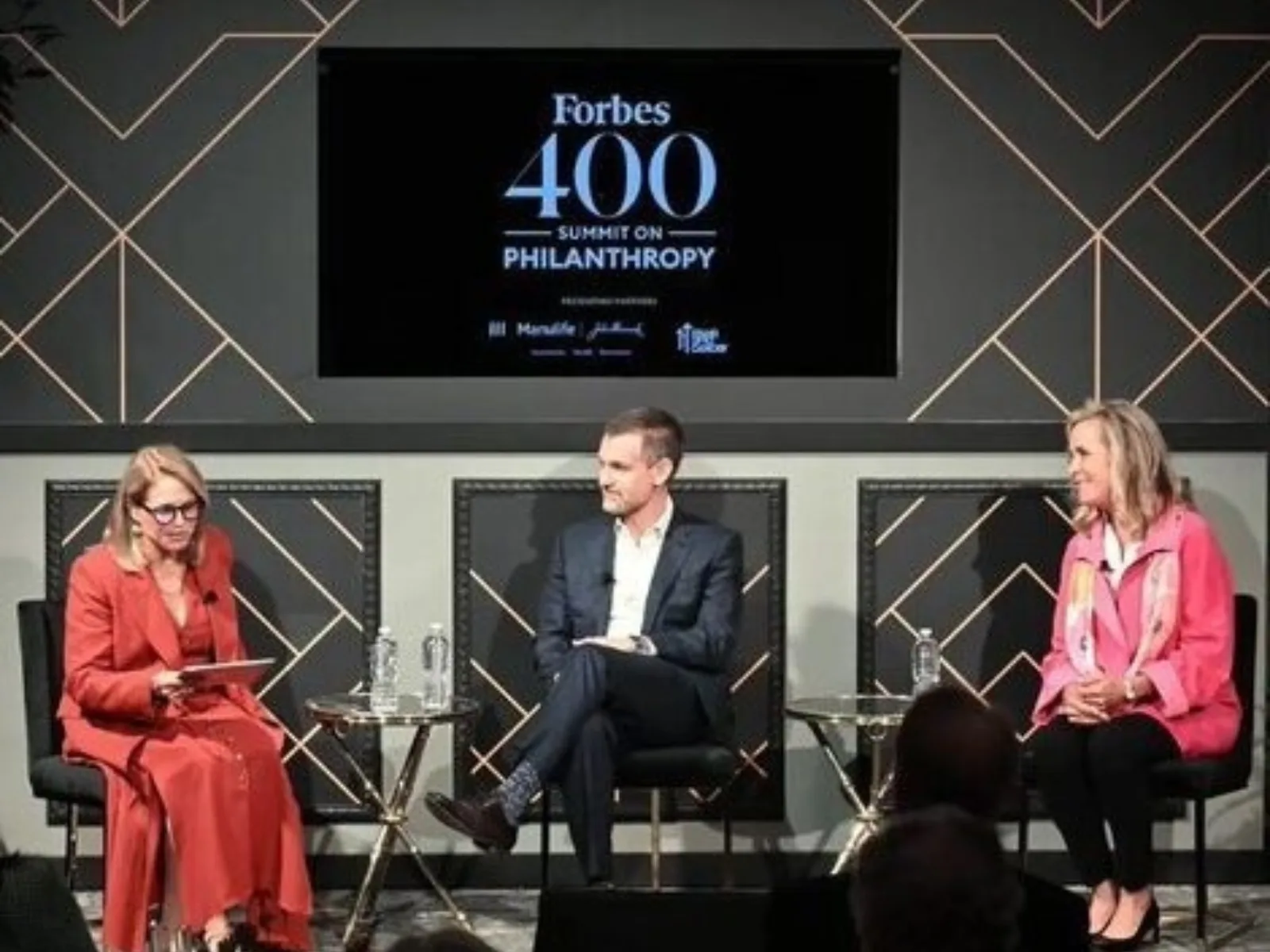
Can We Make Jails and Communities Safer? Evaluating the IGNITE Program: A Q&A with Crystal Yang
Community Safety
Can We Make Jails and Communities Safer? Evaluating the IGNITE Program: A Q&A with Crystal Yang
Q&A
Program Integrity
Arnold Ventures Statement on Bipartisan Bill to End Fraud-Riddled Employee Retention Credit
Higher Education
Degrees of Accountability: Rethinking Incentives and Sanctions in the Higher Education Act
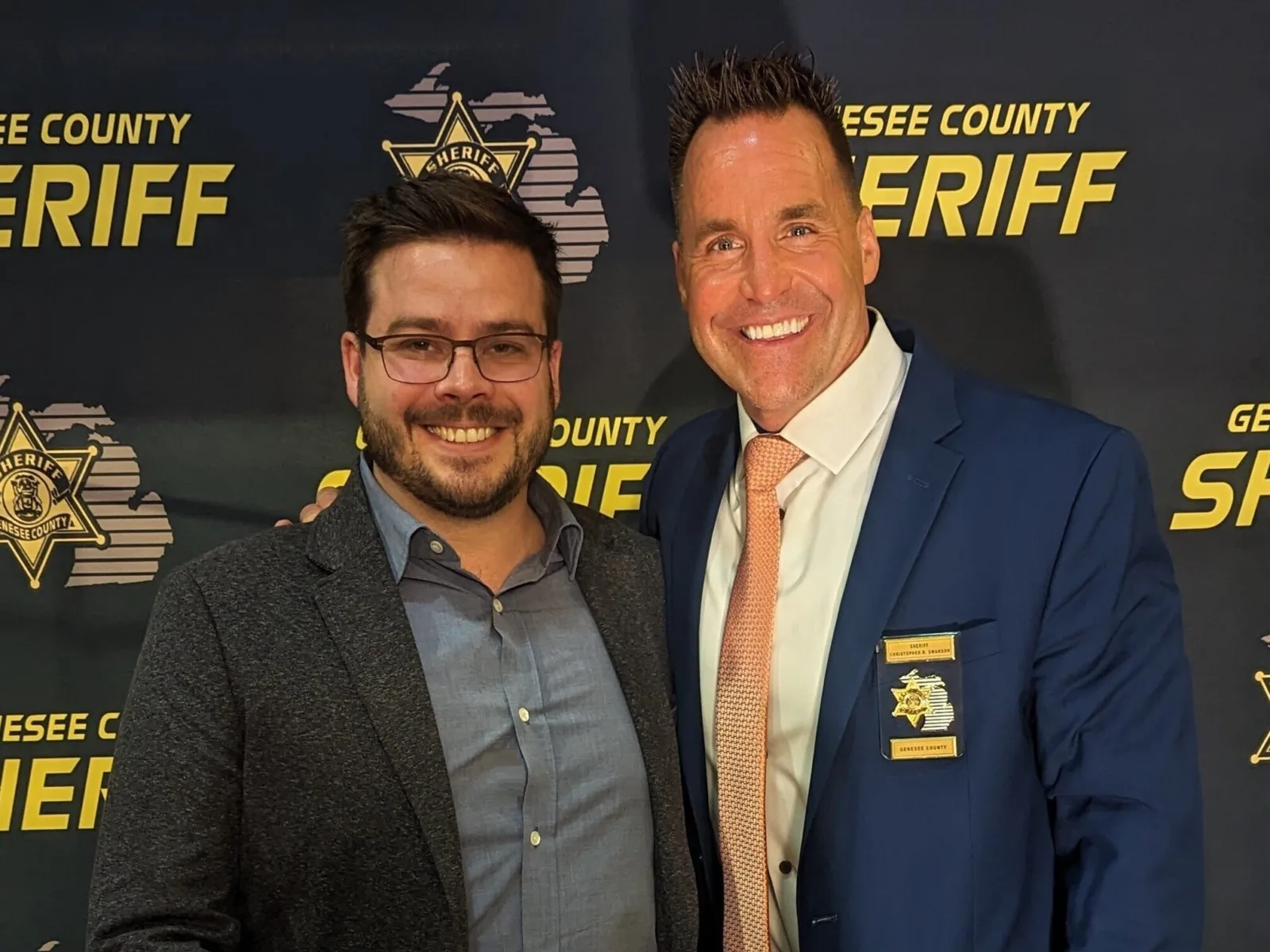
Analyzing the Effects of Electronic Pretrial Monitoring: A Q&A With Roman Rivera
Community Safety
Analyzing the Effects of Electronic Pretrial Monitoring: A Q&A With Roman Rivera
Q&A
Program Integrity
AV Recommends Ways to Improve the Congressional Budget Office
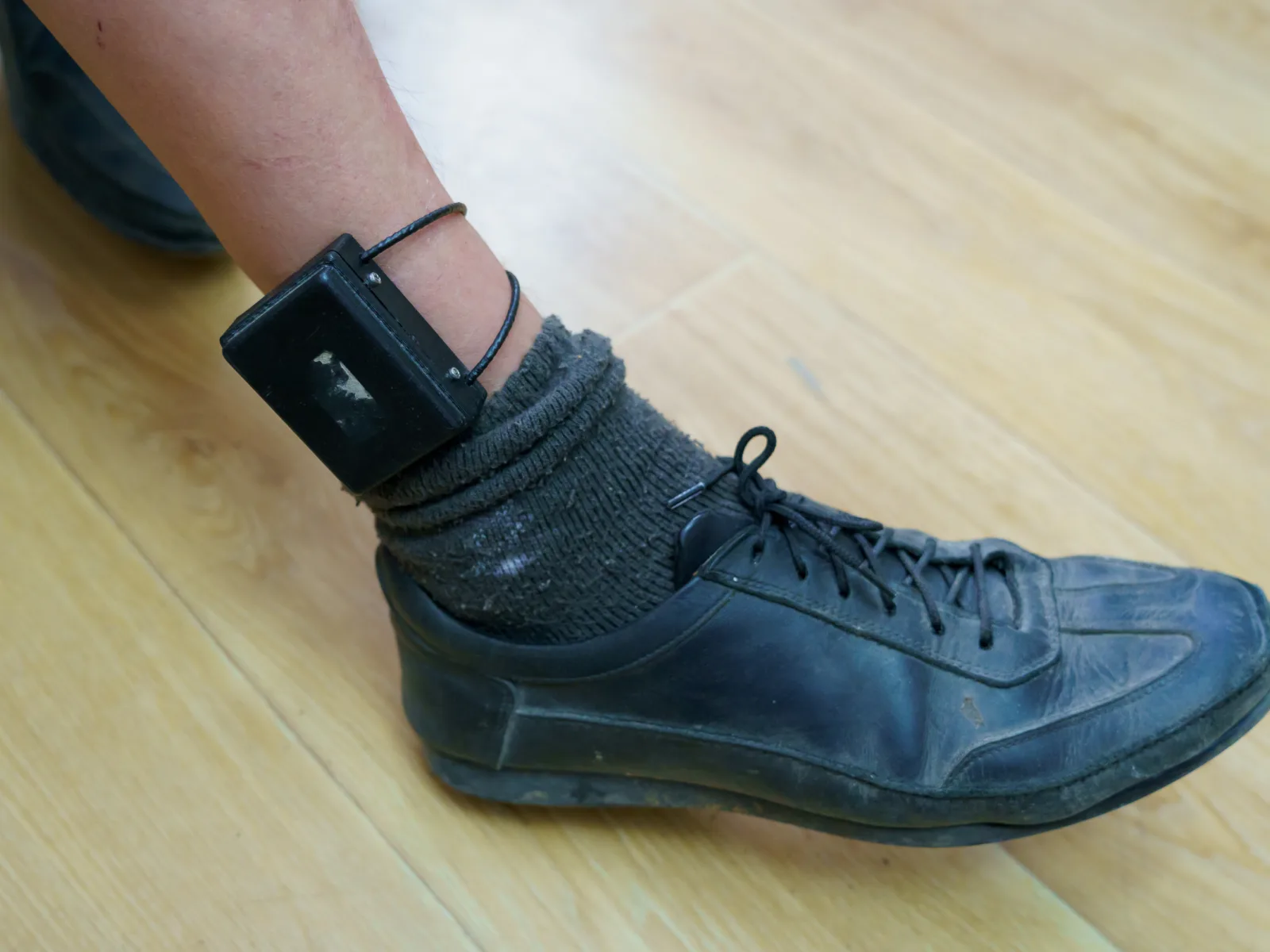
About Us
We strive to maximize opportunity and minimize injustice by investing in systemic solutions that will outlast our funding.