Research shows that a relatively small share of police officers account for a disproportionate number of misconduct complaints. Unfortunately, by the time officers begin racking up civilian complaints, it is often too late to terminate them thanks to stringent union rules. But what if those officers at high risk of misconduct could be identified and weeded out before they joined a department? That’s the question examined in a new research paper by Jens Ludwig, Dylan Fitzpatrick, both of the University of Chicago, and Aaron Chalfin of the University of Pennsylvania.
The researchers discuss what is known about the ability of new “Big Data” methods to capture predictable risk of officer misconduct. Ludwig et al. argue that by collecting more sophisticated data as part of the application process, like better psychological and behavioral science tests, police departments may be able to predict which trainees are most likely to engage in future misconduct. With this new information in hand, departments can then either decline to move forward with the recruit or provide them with additional training.
However, this system comes with its challenges.
Because such “Big Data” analysis is costly and requires great technical expertise, the authors recommend that individual police departments work together with their state government to pool resources and take advantage of economies of scale. While The National Decertification Index tracks decertified officers, a number of states do not engage in decertification practices, and the reasons for an officer’s departure may still be highly relevant for other departments even if it did not result in decertification. Establishing statewide and national databases of officers who may be at greater risk of misconduct would also prevent rejected officers from simply moving to another department.
Algorithms used in the hiring process must also be carefully designed so as not to introduce bias. Nominally objective data used in these algorithms can end up unintentionally reflecting biased outcomes of racist systems. For example, a citation for drug use may seem like a straightforward standard as a risk factor for hiring, but we know that Black people are far more likely to be stopped and cited for low level marijuana possession than white people, even though surveys show both groups use marijuana at equal rates.
Furthermore, people and communities impacted by the justice system often have the least say in how data is collected and utilized, or even what information is collected as data. Without their input, criminal justice data only tells us part of the story. The process of creating algorithms must be inclusive and transparent.
Arnold Ventures spoke with Jens Ludwig about the paper, State Policies and Police Personnel Decisions, which were presented at a 2020 roundtable. Ludwig is the Edwin A. and Betty L. Bergman Distinguished Service Professor at the University of Chicago’s Harris School of Public Policy, where he directs the Crime Lab and co-directs the Education Lab. He also serves as co-director of the National Bureau of Economic Research’s working group on the economics of crime. The interview was edited for length and clarity.
Arnold Ventures
What attracted your interest to the topic of police reform?
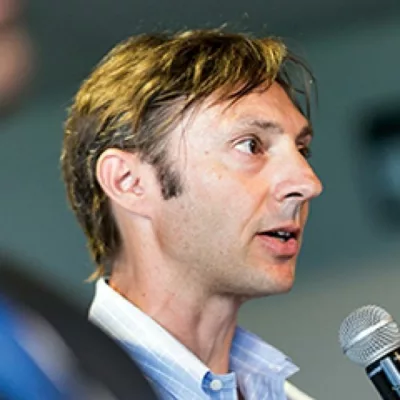
Jens Ludwig
As someone who lives in Chicago, and on the South Side specifically, I can see all of the challenges of policing in America every day up close. Other cities around the country, and other countries around the world, show us that there is a better way we can be doing things. I helped start the University of Chicago Crime Lab in the hope that the power of data and data science could be useful in helping address these sorts of challenges that are facing not just my home city of Chicago but cities all across America.
Arnold Ventures
How does your training as an economist help you evaluate options for improving law enforcement?
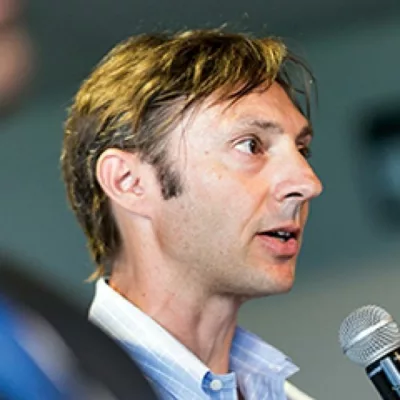
Jens Ludwig
One of the things I appreciate most about economics is how data-driven the field is. That to me seems really important because history is full of policy innovations that turned out to be not nearly as helpful as we all initially hoped, or in some cases even had major unintended consequences. Doing everything we can to use data to increase the chances that our new policies are as helpful as possible right out of the gate, or, once policies are implemented, to any identify needed course corrections as quickly as possible, seems critically important given the enormous needs we have for social progress in so many policy areas — including police reform.
Arnold Ventures
At multiple points in the paper, you mention the lack of national data about police misconduct. Why don’t we have better information about civilian complaints, officer firings, or other potential misconduct?
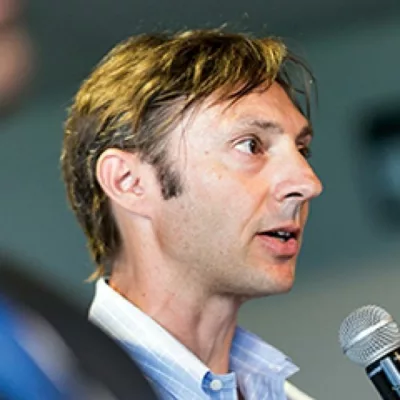
Jens Ludwig
Part of the problem here is that in the America of 2021, so many of our policy debates have become purely political and ideological, so the idea of facts as a key thing to be guiding our thinking and our policy decisions is just not front and center in many people’s minds. We’re not very good right now about investing in data, or paying attention to evidence, in lots of areas of public policy, including in police reform. On top of that, we have a very fragmented system of government in the U.S. where responsibilities are distributed across different levels of government. In a world in which local government reporting of data to the federal government on most criminal justice topics (including policing) is voluntary, how can anyone be surprised that the data infrastructure we have isn’t nearly what we need it to be?
Arnold Ventures
Why is it so difficult to identify officers who are likely to be bad fits for police departments?
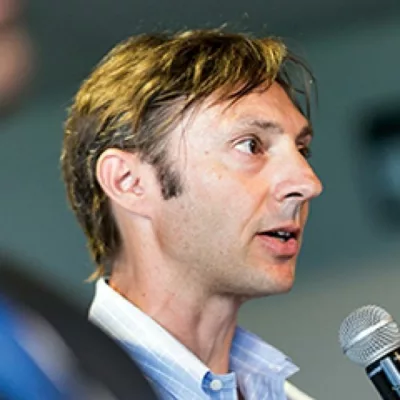
Jens Ludwig
One of the challenges in identifying officers comes from a sort of mismatch between where in the HR pipeline of an officer’s career police department decisions might have the biggest impact on policing outcomes, versus when in the career cycle we can predict future adverse outcomes most accurately. At the initial screening stage, and then at the end of the probationary period, departments have a lot of flexibility about who to hire or not, and so in principle could exert a lot of influence over who winds up becoming a police officer. But at least in the data we’ve been able to look at so far, it turns out to be much more difficult to accurately predict risk of future adverse outcomes at those stages compared to later on in an officer’s career, once the department has more data on each officer and what they’ve been doing on the job. For officers past the probationary hiring stage, our goal then needs to be finding really effective interventions to target and deliver as part of early warning systems or early intervention systems.
Arnold Ventures
How can police departments identify ex ante (before the event) factors for officers in order to either avoid hiring them or provide appropriate training?
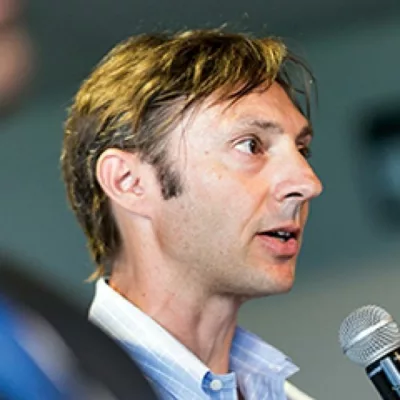
Jens Ludwig
One of the things we’re seeing in other industries and occupations is growing openness to expanding the type of data that are being collected as part of the hiring stage, in an attempt to do a better job predicting what someone will do on the job if they’re hired. If one of the challenges in policing is that the usual information departments collect at the hiring stage isn’t so useful in identifying what an officer will do on the job if hired, it makes you start to wonder if being more imaginative in how we do the hiring process in policing could potentially help to some degree.
Arnold Ventures
What type of data do law enforcement agencies collect during the hiring stage? Is this different from other jobs?
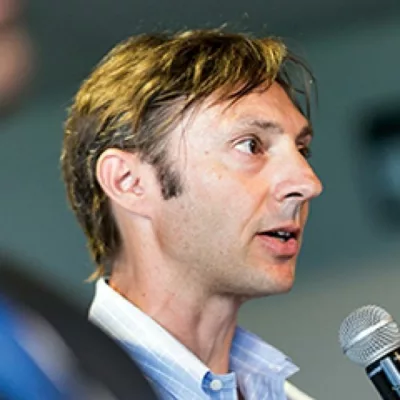
Jens Ludwig
My sense is that the most common feature of the field of policing is variability. Police departments differ enormously in almost everything — how they train officers, how they supervise, equip, support, and discipline them — and I think this includes hiring as well. Most departments will ask some basic pre-screening questions, such as educational attainment, prior work history. Then departments will differ a lot in what additional information they collect, like whether they ask people to do a video exam in which they respond to a video of some scenario. They may also ask applicants to complete psychological, physical, or other tests. Even with all that variation, I doubt there are many police departments out there that are capitalizing on all the things behavioral science has learned about how to help organizations pick the sort of people those organizations are trying to find.
Arnold Ventures
Why is the probationary period for police officers so important, and why is it so difficult to fire them after that period?
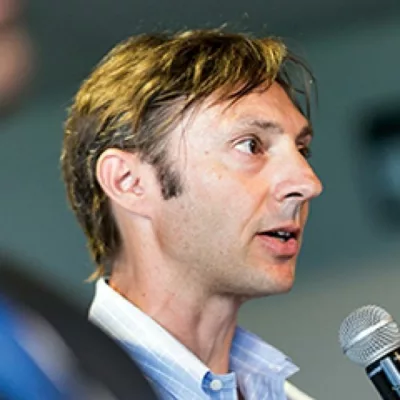
Jens Ludwig
City government is always a balancing act between competing objectives. If you read any of the histories of what American cities were like 100 years ago (or maybe 50 years ago or more recent than that even), you get the sense that lots of government jobs were filled on the basis of political connections — patronage. A new mayor would come in, and they’d clean house and put in all their campaign supporters. Civil service protections were put into place to make it harder to fire people in government jobs, which might have helped reduce corruption and patronage to some degree but also might have the effect of making it harder to dismiss an officer that the city leadership and residents wish would be dismissed. Similarly, public sector unions were initially set up to, among other things, ensure workers had decent working conditions and weren’t fired unjustly. But now we’re seeing debates across the country about whether we’ve gone too far in the direction of making it difficult to dismiss certain public-sector workers. We see the same sorts of tradeoffs and debates play out in other key government jobs — teachers, for instance. Whatever the merits or demerits of the current system, they don’t seem very likely to change in the near future. That makes it important to get better information about how an officer is doing during their initial probationary period, so that departments can decide who to make a permanent officer.
Arnold Ventures
How effective are existing training and behavioral modification programs in improving the performance of officers at high risk of misconduct? How should such programs be evaluated?
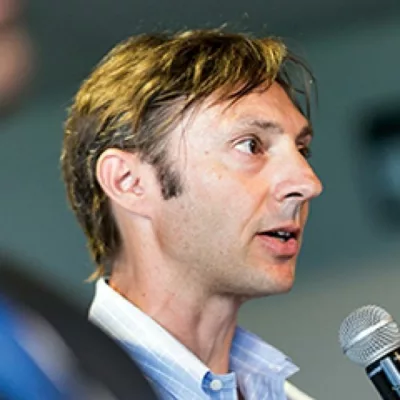
Jens Ludwig
In our paper we talk about how we can try to use data to identify officers who are on a trajectory that puts them at elevated risk for misconduct, with the hope that departments can then do a combination of informing HR where appropriate and providing officers with extra supports and training where that’s appropriate. But these supports and trainings can only work if we really start to get serious about rigorously evaluating the impacts of these interventions. The limited evidence on the effects of these candidate interventions is just part of the larger problem of limited data about what works in policing.
Arnold Ventures
We’re increasingly learning that supposedly objective data used in algorithms can inadvertently perpetuate the biased outcomes of racist systems. How can you ensure that using algorithms to inform hiring decisions doesn’t perpetuate racial bias in policing?
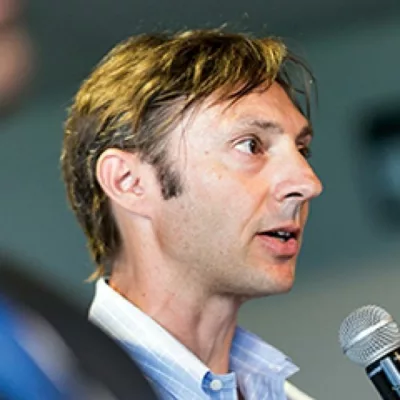
Jens Ludwig
One of the biggest concerns with the growing use of algorithms in public policy applications, including policing, is the very real possibility that the data they rely on may be biased. For example in the case of hiring, a common mistake is to construct algorithms that predict the hiring decisions of past human HR staff — and as a result, wind up automating the bias in those human decisions. I’ve worried a lot about this problem of algorithmic bias and have been doing work on this with Jon Kleinberg, a computer scientist at Cornell; Cass Sunstein, a professor at Harvard Law School; and my University of Chicago colleague Sendhil Mullainathan. One of the things we’ve realized is that algorithms essentially come with what you could think of as an “equity knob” — a well-built algorithm has the potential to offset the bias in the underlying data and achieve whatever other equity goals society might have in a given application. But the potential to use that equity-knob feature of algorithms isn’t capitalized on nearly often enough in practice. Lots of algorithms out there in the public policy space just aren’t being built with those equity objectives in mind. Stepping back, the big-picture problem behind that problem is that existing anti-discrimination laws were built to deal with how humans discriminate. As Jon, Cass, Sendhil, and I have argued in a recent paper in the Journal of Legal Analysis, Discrimination in the Age of Algorithms, the way algorithms discriminate is very different from the ways humans discriminate. We urgently need to update our legal frameworks to account for and address that.
Arnold Ventures
In the paper’s conclusion, you write that “across a wide range of public policy domains we are seeing a movement towards ‘evidence-based policy,’ that uses data to both create new interventions and evaluate their effectiveness.” To what extent are America’s police departments relying on evidence for their practices, as opposed to past practice and institutionalized procedures?
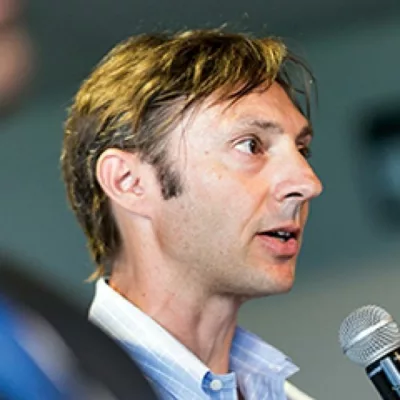
Jens Ludwig
A lot of what police departments and government agencies do tends to be more a function of momentum than deliberate consideration. Getting out of this pattern may require some help with what you could call the “supply” of innovation; that is, most government agencies, including police departments, are so busy trying to deal with their day-to-day operational crises that they don’t have the bandwidth to step back and think about what they’re doing, what they might be doing differently and potentially better. But I think it will also require some change on what you might call the “demand” side of innovation. Part of the problem is that the public doesn’t really know what their police departments or other government agencies are doing, much less how to think about whether the outcomes they’re seeing are “good” or “bad” in any larger sense. I’ve become very motivated to try to figure out ways of not just democratizing access to data, which we’ve been working on, but also to figure out how to democratize the ability to get insights from data, so that the public is better able to hold agencies accountable.